Embrace change, take risks, and disrupt yourself
Hosted by top 5 banking and fintech influencer, Jim Marous, Banking Transformed highlights the challenges facing the banking industry. Featuring some of the top minds in business, this podcast explores how financial institutions can prepare for the future of banking.
Generative AI: Hit the Gas or Pump the Break?
In this episode of Banking Transformed, we delve into the world of generative AI with Ron Shevlin, Chief Research Officer at Cornerstone Advisors.
As data continues to be the foundation of AI applications, we explore the potential of generative AI in financial services, as well as the practical realities and limitations financial institutions need to consider. Moreover, we touch on the competitive and regulatory landscape and how financial leaders can navigate it when adopting generative AI solutions.
In the crystal ball segment of our discussion, Ron shares his vision for the future of generative AI in the financial sector and provides valuable insights into what financial leaders must be doing today to prepare for the AI-powered tomorrow.
Where to Listen
Find us in your favorite podcast app.
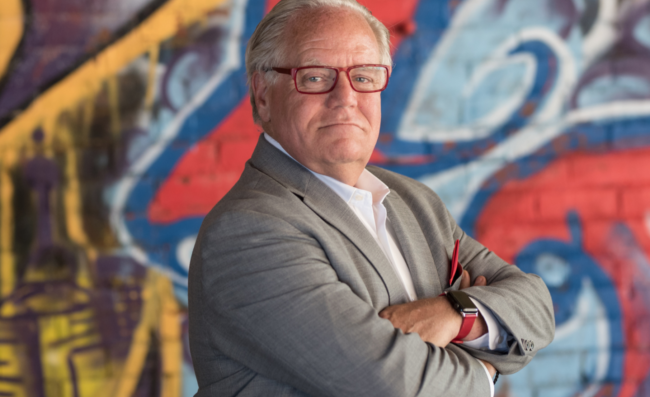
Jim Marous (00:00):
Welcome to Banking Transformed, the top podcast in retail banking. I'm your host, Jim Marous. In this episode of Banking Transformed, we delve into the world of generative AI and ChatGPT with Ron Shevlin, the Chief Research Officer at Cornerstone Advisors.
Jim Marous (00:27):
As data continues to be the cornerstone of all AI applications, we explore the potential of generative AI in financial services, as well as the practical realities and limitations financial institutions need to consider. Moreover, we touch on the competitive and regulatory landscape and how financial leaders can navigate it when adopting generative AI solutions.
Jim Marous (00:50):
In the crystal ball segment of our discussion, Ron shares his vision for the future of generative AI in the financial sector and provides valuable insights into what financial leaders must do today to prepare for an AI powered future.
Jim Marous (01:07):
While there's tremendous promise with generative AI solutions, there's also the need for responsible deployment of all AI solutions in banking. In this episode, we'll discuss the nuances to the hype, while also affirming generative AI's disruptive potential if leveraged thoughtfully over time.
Jim Marous (01:25):
So, Ron, we've been together a couple times in the last few months, and you've been talking quite a bit about AI and generative AI, and I realize that the vast majority of our listeners already know you. But for those who may be unfamiliar, could you share a bit about your background as well as a little bit about Cornerstone Advisors?
Ron Shevlin (01:44):
Absolutely. Hi, I'm Chief Research Officer at Cornerstone. Cornerstone is a consulting firm that serves the mid-sized bank and credit union market, doing a lot of strategic planning, technology payments, and performance improvement work.
Ron Shevlin (01:58):
Having said all that, I don't actually do any of that. I came on board to start a research practice, so I'm generally developing a lot of content and write a blog on Forbes called the FinTech Snark Tank. And I've basically been in the analyst world now for 26 years, starting at Forrester, and then Aite Group, and now with Cornerstone.
Jim Marous (02:19):
Thanks. And it's interesting, I was out of town and couldn't make Money20/20 this year, but you just got back from Money20/20 in Vegas, and I would believe that there weren't any conversations on the event floor or during any of the sessions that did not reference ChatGPT, generative AI or other AI solutions. So, what were some of the key themes you heard around these subject matters?
Ron Shevlin (02:43):
Well, you're right. And it is kind of consuming every conversation, and that's actually good and bad in some ways. I think it's good because it shows a level of awareness for the tools and the promise of them. But on the downside, Jim, the conversation's getting very muddy.
Ron Shevlin (03:01):
It just seems like the terms generative AI, AI in general are not being used specifically enough to get at the various nuances and differences in the types of technology, and that's kind of bad. The other, I think, challenging part from an industry perspective is that with no offense to all the technology vendors out there, simply claiming to be integrating AI into your products and solutions, doesn't really kind of cut it anymore. Because it's not really clear exactly what they're kind of doing.
Ron Shevlin (03:34):
The other point I'd make, and I think this is a good sign, and I've been kind of ... this is a Cornerstone, no pun intended to the presentations that I've been given at conferences, is that at the individual level, I hear from a lot of executives that their people are coming to them and saying, "Hey, are we going to see layoffs because of AI?"
Ron Shevlin (03:54):
And I think there's a growing level of awareness that the real impact, at least in the short-term, and the short-term being five, seven years, throughout the rest of this decade, is that the real impact of a lot of these tools and technologies is really more about productivity improvement and creativity enhancement for that matter than it is job loss.
Ron Shevlin (04:16):
I don't think we're anywhere close to being able to do mass layoffs in the industry because of these kinds of tools. But really, I think there's a general awareness that this is really about major productivity improvement.
Jim Marous (04:32):
It's interesting you talked about the definition and gosh, if banking doesn't get involved in so many things that you try to put into a nice little package when it's not that easy to do. Can you start with explaining a little bit about what generative AI is, how it differs from ChatGPT, how it differs from large learning models and other AI technology in the context of financial services?
Ron Shevlin (04:57):
Yeah, sure. So, start picturing a decision tree, and at the very top of the tree is AI. And under that are various different types of AI technologies like conversational AI, machine learning, robotics, vision, capabilities, things like that.
Ron Shevlin (05:18):
Generative AI is just another one of the types of AI in that row of the tree where the definition, I don't know, there might be like a formal definition to it, but think of generative AI as AI technology that generates various types of output, like text, music, code, data, synthetic data.
Ron Shevlin (05:44):
I'm sure I'm missing a bunch, but think about it in terms of various types of output that it produces. And think about it differently in terms of machine learning, where the output is not necessarily text, although it might be even the form of text. But you're really talking more about quantitative stuff when you're thinking about machine learning and obviously things like robotics and visioning and other types of things don't have a similar type of output.
Ron Shevlin (06:16):
Now, under the banner of generative AI would be tools like ChatGPT. So, ChatGPT is a form of generative AI, but ChatGPT itself, it really only produces text. It actually integrates with other tools, often ChatGPT plugins to produce things like music or code or art or synthetic data and so forth.
Ron Shevlin (06:45):
Now, what forms ChatGPT and products like that are things called large language models. And they're called large because they literally have billions and billions of pieces of input. And that's my best Carl Sagan impression, and I won't do any more impressions for the rest of the show.
Ron Shevlin (07:07):
So, there's this tree and what's emerging is not just ChatGPT, it's not the only large language model out there. In fact, there are many. Then you get sort of the, well, some are more specialized than others, and some of them are open source and some of them use proprietary data as their input.
Ron Shevlin (07:27):
Microsoft a couple of months ago launched their large language model, it's called Gorilla. Facebook has their model, it's called LLaMA, then there are specialized ones. Bloomberg came out with one, probably called BloombergGPT, for all I know. So, there's a lot of them coming out Salesforce. I expect if the most major tech vendors don't have one out now, they'll have one out within a year.
Jim Marous (07:57):
It's amazing to think that the birth of ChatGPT at least in the public, is still just a little short of a year ago. So much has happened and Cornerstone Advisors among other organizations are continually looking at the potential applications for generative AI solutions.
Jim Marous (08:17):
So, what are some of the most promising potential applications for generative AI that the team at Cornerstone Advisors have identified? And where could it possibly had the biggest impact in the short-term?
Ron Shevlin (08:30):
So, it has an impact. Let's look at this from the perspective of a financial institution, Jim, if we can, from a bank or credit union, is probably the easiest way to talk about it, I think. And the potential applications and use cases are actually all over throughout the organization, but where things are really going to be different is that, look, I think the industry has spent the better part of the last 5, if not 10 years, focused on digital transformation.
Ron Shevlin (08:58):
And for I think a large extent, a lot of those efforts have focused on the high transaction volume or high-volume interaction type processes, like account opening, loan origination, customer support. What about the rest of the organization? What about all the knowledge workers? What about the people in marketing, the people in compliance, the people in legal, the people in IT? What have they gotten out of this digital transformation and AI world? Not a lot actually until now.
Ron Shevlin (09:35):
So, there are use cases, I'll give you some examples of use cases that financial institutions have been doing that kind of caught my eye. One bank has kind of redesigned one of their product pages using ChatGPT and plugin tools.
Ron Shevlin (09:50):
The tools identified the right search engine, terms to use, identified the right graphical images to use, actually created a form, and actually I think it was either two to three minutes or two to three hours I got to go look that up, refresh my memory on that one, how long it actually was that it took the tools to create a new webpage that would've taken two to three people, two to three weeks to do.
Ron Shevlin (10:16):
Another example, is HR using ChatGPT and other generative AI tools to connect on LinkedIn and find potential candidates for open positions and actually reach out and recruit them and carry on conversations.
Ron Shevlin (10:36):
So, think of it as a proactive chat bot versus a reactive chat bot, but it's a lot more intelligence in that it's actually finding potential candidates. Chris Nichols, who runs the innovation group at SouthState Bank, has written a lot about this and a lot of great examples coming out of SouthState.
Ron Shevlin (10:54):
One of the ones that I thought was amazing is they used a tool called Auto-GPT, which is a plugin that connects to a paid version of ChatGPT versus APIs. And what it does is it makes ChatGPT autonomous, or at least semi-autonomous.
Ron Shevlin (11:14):
And what SouthState Bank did was use these tools to basically execute a marketing campaign for health savings accounts. And so, over the period of about three weeks, what Auto-GPT did was first connected itself to an email marketing program, created two different versions of an email offer letter, and then went out into the market having connected to the mailing list that the bank has and tested out these various offers.
Ron Shevlin (11:51):
After a few days, they had enough responses to see which one was sort of the champion, which one was the challenger. And then over the period of another week or so, ran more tests of offers to optimize how to personalize the offers, how to determine what the best incentives were in terms of not just price and fees, but things like time restraints and constraints.
Ron Shevlin (12:16):
It learned autonomously what the best personalization elements were. And then after literally 22 days had raised more than $2 million in HSA deposits through their tests. Now, the thing I hate to admit, but it's the honest truth of the matter is, is that this was all a simulation. And I can only imagine it was only a simulation because who the hell is going to trust AI right now?
Ron Shevlin (12:49):
Who knew with all the errors, the hallucinations, the decline in performance between ChatGPT 3.5 and 4.0, you've got be a little bit crazy to just really put this out into the public right now, who knew what any of this was to produce.
Ron Shevlin (13:05):
So, most of the financial institutions that I've seen do it, are still in test and experiment mode versus turn the switch on and then let the damn thing run. And by the way, Jim, this is the tip of the iceberg on the number of use cases that are out there for this.
Jim Marous (13:26):
And it's interesting because as you said, everybody's testing the waters and we're ... some of this imagining, some of this is testing things. I mean, I'm testing it every day, and unfortunately every day I test a new model, there's another tool that comes out that says, "Oh, this one can help you build presentations, or this one can improve your emailing." And it's another platform.
Jim Marous (13:48):
And you go, "I can't handle seven or eight brand new programs. I can hardly handle PowerPoint, Word and Excel. So, I only have so much brain power left." So, from your perspective, and because we're really at the beginning point of all this, how do you see generative AI transforming the front end experiences? I know you gave some examples, but where do you see it to being deployed, maybe at scale and specifically to really improve the consumer side of the experience?
Ron Shevlin (14:21):
Jim, think about the user experience. And this is not just new to AI, but think about what the customer journey is, so much generically. It's four pieces, the acronym is fend, if you need an acronym to help you remember things. So, what do we do?
Ron Shevlin (14:42):
First is we find providers of a particular product or service, then we evaluate them, then we may or may not negotiate prices, fees and things like that. And then we deploy or buy. Now over the past 25 years, basically since Google came out, maybe even Yahoo back in the day as well, we've absolutely transformed the way consumers find providers.
Ron Shevlin (15:08):
At the other end of the journey, the past 10 years, we still got a long ways to go. You constantly harp on this to financial institutions, rightfully so, but they have done a better job at the account opening side, both from a deposit and other types of products and loans and things like that, loan application, digital loan application. So, they're getting better on that.
Ron Shevlin (15:33):
But we still have a big hole in terms of how well these tools help us evaluate the existing tools, the existing sites and tools and things like that. Google has tried some things to help evaluate vendors. They actually took it off the market, it wasn't that good.
Ron Shevlin (15:51):
And really there's been nothing out there to help negotiate, optimize, that type of thing. Some things are kind of emerging, but it's in that middle piece of the journey, Jim. It's the evaluation and negotiation. And it's interesting because for a lot of us, we're not comfortable negotiating.
Ron Shevlin (16:11):
And I don't just mean car prices with the car dealer. I mean, call on the bank and go, "I'm giving you a lot of money, give me 2.5 instead of 2%, or give me a better rate on the mortgage or do all of this." But there's a great example I like to use in a lot of my presentation.
Ron Shevlin (16:29):
There's a guy, he's the CEO of a company called DoNotPay. And let's get real, this is not your typical consumer. He's a programmer, he's a technologist and he's the CEO of a technology company. And I think it was pretty much a publicity stunt, but he went out and said, "I'm going to turn over the management of my financial life to generative AI tools."
Ron Shevlin (16:53):
Well, the first thing he did was one of those, do not try this at home things. He linked all of his accounts to generative AI tools through plaid APIs. Not a thing I'd recommend to anybody, and I'm certainly not going to do it myself, so it was a marketing stunt.
Ron Shevlin (17:10):
But after having done that, he then told the tools, "Alright, go out and find me a subscription that you can cancel." And it came back and said, "Well, we see you've got a Planet Fitness, do you go?" He said, "Cancel that." And it went out and basically took care of the cancellation of the subscription for him.
Ron Shevlin (17:30):
Then he told it, "Go find me an expense that I have incurred that you can get a refund for." So, it came back and said, "Well, we see that you flew from New York to London and paid $35 for the Wi-Fi, did it work?" He said, "Nope."
Ron Shevlin (17:46):
And so, not only did it take care of getting the refund, but it was interesting in how it did it. It wrote a letter, an email letter to the airline and actually cited the FCC regulations for unfair sales practices that you and I would probably never even thought of including in the letter, and it got him the $35 refund.
Ron Shevlin (18:09):
And then he said, "Okay, go look at my bills and the expenses and find me some money that you can get back." It came back and said, "We found $50. We think we can get you off of your Comcast bill, is that good?" He said, "No, go get more." And came back with another $50. So, it got him $100.
Ron Shevlin (18:27):
So, Jim, think about the change in the customer experience now. Back in the days before a lot of our listeners here were even born, guys like you and I on a Saturday would groan and gosh, shit I got to spend the whole day calling insurance agents to find the best price for our car insurance.
Ron Shevlin (18:49):
And then Google completely transformed that because we could find all the players within five minutes, but we still couldn't do a good job of evaluating, and we didn't do a very good job if we even did any negotiation at all. And now of course, we can apply for all of that stuff online, but these AI tools, Jim, are taking over the total process for us.
Ron Shevlin (19:12):
And there is a picture that I use in my presentations and it's a picture that just draws a line between those folks who are over 50 and those who are under 50. It's a picture of Rock 'Em Sock 'Em Robots. Remember that? Rock 'Em Sock 'Em Robots.
Jim Marous (19:32):
Oh, yeah.
Ron Shevlin (19:33):
And I use that picture to say, "This is where we're going. It's going to be your bots, the provider's bots versus our bots, the consumer's bots." And hey, got to give credit to Brett King. He wrote about this, what, seven, eight years ago and augmented. Everyone's going to have bots and I think-
Jim Marous (19:53):
Their personalized bot, exactly. It's interesting because what you get into there, it's the value proposition as opposed to simply cost. So, it will start to learn what you value and what you put to a high level, which makes it so generative AI will actually work because a consumer's going to be willing to give up information if they think they're going to get something of value in return.
Jim Marous (20:17):
And again, it's not necessarily just dollars and cents, it may be time savings, it may be trust, it may be other elements. But as you mentioned, the insurance example, I can tell you that I threw up my hands and just gave up on the whole Medicare situation.
Jim Marous (20:31):
So, I said, "There's just no way I can figure this out," my wife took the process over. But it would be really a great use of generative AI and AI tools to help me make the decision as what's the best combination for the type of insurance I need, dental or eyesight or whatever it may be. And get me not just the best rate, but a well-rated organization that can provide the ability to get the add-ons for the insurance policy.
Jim Marous (20:58):
So, outside of the experience, organizations are really grappling with legacy systems and infrastructure. How do you think generative AI will be integrated into existing technology stacks to improve the back office functions?
Ron Shevlin (21:16):
Well, there's two ways. I think over the next five to seven years, and probably even a little bit longer than that, you're going to see the providers of a lot of those process or functional area, functional applications, integrate these kind of capabilities into the core, into the ancillary systems, there's no question about that.
Ron Shevlin (21:39):
But they'll still be instances of these technologies and tools that just access through APIs and access the data stores separately and won't be necessarily integrated into the core and into the ancillaries. Jim, I think the big issue and question for a lot of technology providers and for financial institutions in particular, is whose bots, whose platforms are consumers going to use to negotiate with the provider's bots and platforms?
Ron Shevlin (22:14):
If you're a JPMorgan Chase customer, you don't want a JPMorgan Chase bot negotiating with JP Morgan Chase, that doesn't make a lot of sense. And you raised the issue about trust. So, this is where the vendors are all saying, "Oh yes, well, we've got AI integrated today." I'm not so sure.
Ron Shevlin (22:35):
One of my colleagues, John Meyer, not too long ago, the week before last, had a post on LinkedIn where he said, "Yeah, if vendor tells you this, here are the six or seven questions, whatever it is, to ask them to verify that great thing, go find that. For everybody listening, go take a look at those questions. Because that's what you want to ask vendors to make them prove that they really have."
Ron Shevlin (22:57):
But five years down the line, maybe even a little bit longer, maybe a little bit shorter for some of them, they will get there with truly integrated, but it still is going to be a standalone tool for a lot of people within the financial institutions.
Jim Marous (23:13):
That article you mentioned. It's on your LinkedIn site, by the way. Anybody goes to Ron Chevlin on LinkedIn, will see what he's referencing. There's actually, I think, nine questions that you should ask. Because you're right, you go up and down the floor at Money20 last week, and you realize that every single organization is saying the same thing.
Jim Marous (23:34):
We have brand new generative AI tools that were deployed against our solutions. And it's crazy because people can use that quote and they could prove that they're doing it, but there's massive differences in the levels of doing that. And that's going to take some time to shake out who's actually deploying it in the way that I want it deployed as a partner. So, let's take a short break here and recognize the sponsor to this podcast.
Jim Marous (24:06):
Welcome back to Banking Transformed. So, I'm joined today by Ron Shevlin, Chief Research Officer at Cornerstone Advisors. We've been exploring whether financial institutions should hit the gas and embrace generative AI or pump the brakes a little bit and proceed with caution.
Jim Marous (24:20):
So, Ron, right before the break, we talked about the questions you should probably ask your solution providers. They say, "Oh, we have generative AI tools." Going beyond that, going beyond what the vendor may be trying to sell you, another concern that every financial institution is worried about is bias and fairness. How are financial institutions trying to work to ensure that these AI models will actually be ethical and free of bias?
Ron Shevlin (24:50):
Well, first of all, let's make sure we're talking about the same thing, Jim, because so far in this discussion, we've really been focused a lot on generative AI tools and technologies. And I recently moderated a panel discussion at Boston FinTech Week, which by the way, is a great event everybody should be going to next year.
Ron Shevlin (25:15):
The topic of discussion was generative AI. And one of the panelists started raising issues and concerns around bias and fairness and all of that. And at that point, it kind of hit me, wait a second, are we talking about machine learning or are we talking about generative AI?
Ron Shevlin (25:32):
And I had another discussion with other folks, and I said, I don't think that the fairness and bias issue is as built in to generative AI tools as it is machine learning type approaches. Because I mean, that's generally focused on things like lending type things.
Ron Shevlin (25:53):
But on the other hand, let's go back to the SouthState Bank example of the marketing campaign that it ran. And I'm sure that one of the reasons it did not actually launch that in real life versus just through virtual and attest, was that there could have been bias in terms of the types of offers or the language that was used, or the level of personalization or the elements of personalization that were incorporated in there.
Ron Shevlin (26:27):
So, look, the key thing from a financial institution perspective with all of this is that the root cause of any of this bias or any potential bias in unfairness is the data. And I don't know that there's any easy answer to doing this.
Ron Shevlin (26:48):
Now, how are firms doing this? Well, there's an emerging number of companies that are coming out under various names. One of the labels, I kind of like, and we'll see if it sticks, is fairness as a service. Of course, everything is, as a service these days, but there are a couple of firms that come under that banner. I'm forgetting the names of some of them.
Ron Shevlin (27:09):
Uplink is a great example of companies that are doing that and ensuring that there's a broader set of data that is infused into the decision-making process. But again, it's not clear to me that that's as much of an issue with generative AI as it is with machine learning that's fueling a lot of the underwriting and lending decisions.
Jim Marous (27:35):
So, we were in Nashville together a few weeks ago, and in your presentation you started with the whole concept or the discussion around whether or not generative AI or AI overall would replace you, me and everybody else who's listening to the podcast. What was your takeaway from that?
Jim Marous (27:53):
Because I know, we look at how we're using, you go, "Geez, this is getting closer and closer to the way I do things," but do you see this really, at least in the near term, being something that's going to replace workers, enhance workers, or actually add jobs?
Ron Shevlin (28:13):
Putting my money on productivity and enhance. Jim, I'm fond of saying over the past couple weeks that ChatGPT is to 2023, what Lotus 1-2-3 was to 1983.
Jim Marous (28:29):
For those of you who were born after 1983, it wasn't fun. Yeah, but scary.
Ron Shevlin (28:34):
Yeah, and I say that in presentations and generally I get the eye roll. It's like, "Oh God, here's the old guy telling me about the old days again." But there is I think a valid reference here in that look, when Lotus 1-2-3 was released, and by the way, as you know, it was not the first spreadsheet on the market that honor went to VisiCalc.
Ron Shevlin (28:56):
But when Lotus was introduced 1-2-3, it immediately gained a huge amount of adoption and utilization. And what it did was automated a lot of manual stuff that people were doing. We could finally put down our HP calculators and how to write down all those numbers, but people actually used Lotus 1-2-3 to do things like database management. And believe it or not, they actually used it for word processing as well.
Ron Shevlin (29:26):
But there were some problems. There was a lack of quality. People would hard code errors into formulas. There was very little documentation and transparency into the spreadsheets. And there was also very little consistency in how the tool was being used within an organization.
Ron Shevlin (29:42):
And that's very similar to ChatGPT today. There's a lot of errors because of hallucinations, very little documentation, and transparency into what was being asked and what are the sources of information, which of course, leads to a lot of these hallucinations and very little consistency in terms of how the tool is being used today.
Ron Shevlin (30:05):
But just as Lotus evolved to start to incorporate a lot of the plugins that were available, I think that's what will happen with ChatGPT and some of the other competitors that are emerging. And so, for a good period here of at least 5 to 10 years at least, what we're looking at is a lot more about productivity enhancement than job loss.
Ron Shevlin (30:30):
But I think there's another aspect to this, Jim, that is really super important. It's not just productivity enhancements, it is about creativity enhancement. It is about getting to new things faster. And our friends JP Nichols and Jason Henrichs who've done a lot of work with innovation over the last few years.
Ron Shevlin (30:51):
When I started writing about this, I reached out to Jason and I said, "Look, you're going to hate me for this, but I can handle it." And I said, "This innovation stuff is BS. All this going out and trying to juice up the creativity and let's do some innovation exercises," Jim, total waste of time.
Ron Shevlin (31:09):
When you can go to a ChatGPT and say, "Give me some ideas about this." And the ideas are never implementable and deployable, but what they are, are spurs to make you think about what's potential. My wife is always on my case, "Hey, why don't you get ChatGPT to write your blog posts?" I'm like, "Well, it wouldn't be my blog post."
Ron Shevlin (31:30):
She goes, "Well, then tell it to write it in the style of Ron Shevlin." It doesn't work. But what it does do when I ask it that is it gives me ideas. And it's not just about artistic ideas and things like that, the innovation is about problem solving.
Ron Shevlin (31:47):
And by having better access to the disparate data sources in an organization, I think it's going to help people be more creative about problem solution and getting their jobs done. So, definitely see this a lot more in the next 10 years as a co-pilot than as a — it augments us, it does not replace us.
Jim Marous (32:11):
It's very interesting, you should say that because I've done the same thing with my Monday articles for The Financial Brand. And what I found was it eliminates writer's block. It's amazing how it does it, because if you get a starting point, you then can write around it and you say, "Oh, geez, I missed that little thing that was around the corner that I may have not have brought up. Or, geez, the thing I was thinking was not nearly as important as I look at it from another perspective."
Jim Marous (32:37):
So, very much like you would say, "Hey, try to see if you can't write me the article on this, that, or the other thing." And somebody comes back and you go, "Okay, it's not me. It's not the way I would put out." But you brought some very good points here, and that's where it's important.
Jim Marous (32:50):
Also, the ability to combine insights from multiple sources. There's a thing called Claude.ai that can take multiple PDFs from across the industry, look at it, pull the major insights out and provide it to you. Now, that helps me.
Jim Marous (33:11):
Because what that does, it speeds up the process of what I do in my writing, but it's still me writing it. I also use for podcasts, I'll say, "I'm interviewing Ron Shevlin on generative AI and ChatGPT, give me 10 questions that you think I should ask," and I'll ask multiple generative AI platforms, the same thing.
Jim Marous (33:32):
What it does, 8 out of 10, 7 out of 10, or once I would've asked, but there may be one, two, or three that not only are maybe a brand-new question, but a better wording question. So, again, it's looking and saying, "Okay, I wouldn't mind another set of eyes looking over my shoulder.
Jim Marous (33:49):
So Ron, we talk about this all the time, and we've had debates about it. We've had sessions on it where we've worked together in a pardoning the interruption at MX two years ago. We as an industry and as executives, tend to sometimes go for the next shiny object.
Jim Marous (34:06):
And it's not like financial institutions need another priority because they have so much on the table. How does generative AI and ChatGPT work and fit into the list of immediate priorities that financial institutions have today?
Ron Shevlin (34:24):
Jim, financial institutions are focused a lot right now because of the economic situation on cost reduction. And I do think that there is an opportunity to reduce cost. I don't actually think it's necessarily through firing people and payroll reduction as it is in reducing third-party expenses that are being incurred today.
Ron Shevlin (34:49):
For example, ChatGPT with some plugins can do a good job of creating code, debugging it. One financial institution was using it to port data from one data model to another, something that probably would've taken him half a million or maybe even a million dollars to do through consulting services. And we're able to do it with about $1,500 worth of ChatGPT expenses.
Ron Shevlin (35:13):
So, it's about not firing people to get the job reduction, but finding out we are aware that third-party expenses, and I can only imagine my boss is hearing this now and going, "Wait, you're telling people to use ChatGPT to reduce consulting expenses?" I'm like, "Yeah, but not our consulting revenue, other firms consulting revenue."
Ron Shevlin (35:38):
But that's where the opportunities are. It's about making people faster and better. And so, there's this opportunity to kind of reduce expenses, but it isn't in term, it's not from reducing headcount. That's not where I think the cost savings are going to come from. But it's a productivity enhancer. And that's where a lot of firms should be looking to figure out how do they make the best use of it.
Jim Marous (36:09):
And finally, one thing you're known for, something you take great pride in, I know, is to bring practical solutions to the marketplace. You get snarky about it at times, but you call bullshit on things. So, looking into the crystal ball, what do you think the future holds for regenerative AI and financial services? And more importantly, what should financial leaders do today to prepare for it?
Ron Shevlin (36:35):
Jim, thanks for teeing that up. A lot of the hype around this uses the term revolutionary and game changing and all of that kind of stuff, and I guess that makes for good press. But I actually really see this as very evolutionary building on a lot of things that have happened.
Ron Shevlin (36:52):
Look, AI has been around for what, 30, 40, maybe even 50 years at this point. And a lot of the true experts in the technology, and I say true experts, because somebody asked me at a conference recently, "Oh, you're going to talk about AI, you're an AI expert?"
Ron Shevlin (37:06):
And I'm like, "Well, actually no, I'm not an AI expert, but I'd like to think that I'm pretty good at understanding the impact of technology on business, and that's the angle I take." But one of the things that the true AI experts don't get is that the reason there's so much hype today is because we needed what has happened over the past 40 years to set this up.
Ron Shevlin (37:30):
And so, there have been waves, sorry, waves of productivity improvements over the last 40 years that started with the introduction of PCs. And you remember back then, there were a lot of executives who said, "I'm never going to touch a keyboard." Now everybody touches a keyboard.
Ron Shevlin (37:46):
But what a lot of folks don't remember is that when PCs first came out, they were actually not connected. There was sneaker wear and was sneaker wear because we used to take floppy discs from one PC and go run it over to another PC, stick it in there to load up the data.
Ron Shevlin (38:04):
And then local area networks came around and they tied them together, and we gained a new level of productivity. Then the internet came around, and when we basically went from local area networks to one big global area network but, we were still missing a lot of data because a lot of stuff happened outside of the home and outside of our desks. So, that's when mobile devices really became a source of new data.
Ron Shevlin (38:30):
And now we've got generative AI tools that are drawing upon all of the technologies, the tools, and more importantly, the data that have been generated by all of these realms and waves of productivity. So, is it revolutionary and game changing? Yeah, I guess if you want it to be, but I really think this is an evolution in productivity enhancement.
Ron Shevlin (38:54):
And what leaders have to do today is twofold. Number one, figure out and understand, where the shorter-term and longer-term opportunities are for not just process improvement and process enhancement, but job productivity, individual task productivity from a knowledge worker perspective and just as importantly, providing the bumpers, the guardrails for the use of these tools.
Ron Shevlin (39:25):
Because A, you need good data to drive these capabilities. But you have to be careful about what data is being used and how it's being used. And that, I think is one of the big breaks on all of this right now, is that a lot of executives are concerned with, "Well, we don't want our data uploaded to ChatGPT, blah, blah, blah, blah, blah." And that's a valid concern, although it's still not even clear to me whether or not ChatGPT saves any of that data or uses it.
Ron Shevlin (39:55):
But determining governance policies, prioritization, and figuring out the balance between stepping on the gas pedal and stepping on the brakes, I think that's the big challenge that a lot of executives are going to face over the next five years.
[Music Playing]
Jim Marous (40:14):
It's funny, I was going to ask you that question saying that reference our headline on the session, our episode, do you hit the gas or do you pump the brake? But I think what you just said was more important than either one of those is use the GPS system. Use the data that's available to you to get to your destination as efficiently as possible, and that sets your priority.
Jim Marous (40:37):
So, Ron, thank you so much for being on the show. As you know, you're our most popular guest. We've had you on the most time of any other guest. And you have some of the top episodes we've had both on YouTube as well as on the podcast channel. So, thank you so much for spending some time and give us a little bit of update on what happened at Money20/20.
Ron Shevlin (41:00):
Absolutely. Glad to be here, Jim.
Jim Marous (41:03):
Thanks for listening to Banking Transformed, the top podcast in retail banking and the winner of three international awards for podcast excellence. We appreciate the support we have received to make this endeavor a success. If you enjoy what we're doing, please take some time to show some love in the form of a review or maybe five stars.
Jim Marous (41:23):
Finally, be sure to catch my recent articles on The Financial Brand and the research we're doing for the Digital Banking Report. This has been a production of Evergreen Podcasts. A special thank you to our senior producer, Leah Haslage, audio engineer, Chris Fafalios and video producer Will Pritts.
Jim Marous (41:41):
If you've not already done so, remember to subscribe to Banking Transformed on both your favorite podcast app and on our YouTube channel for more thought-provoking discussions at the intersection of finance, technology, and innovation. Thank you for joining us, and until next time, keep innovating and transforming.