Embrace change, take risks, and disrupt yourself
Hosted by top 5 banking and fintech influencer, Jim Marous, Banking Transformed highlights the challenges facing the banking industry. Featuring some of the top minds in business, this podcast explores how financial institutions can prepare for the future of banking.
Why AI and Machine Learning is The Future of Bank Differentiation
Data and advanced analytics lie at the core of every financial institution wanting to build stronger engagement capabilities. Unfortunately, many organizations continue to struggle to apply data that will improve the customer journey.
To succeed, banks and credit unions have to understand and take action — in real time — to the consumer opportunities that data reveals.
On today’s Banking Transformed podcast, we have Nate Shahan, co-founder and chief product officer of Segmint. He will share how financial institutions can build business cases today that will help fund data and analytic investments in the future.
This episode of Banking Transformed is sponsored by Segmint
Segmint empowers financial institutions and financial technology providers to easily understand and leverage data, interact with customers, and measure results. Derived from billions of transactions, Segmint provides the fastest and most accurate customer insights through advanced data tagging, categorization, and contextualization. Our insights enable all functions of an organization to inform strategies including competitive analysis, risk, marketing, customer experience, and product innovation.
Visit HERE to learn more about Segmint.
Where to Listen
Find us in your favorite podcast app.
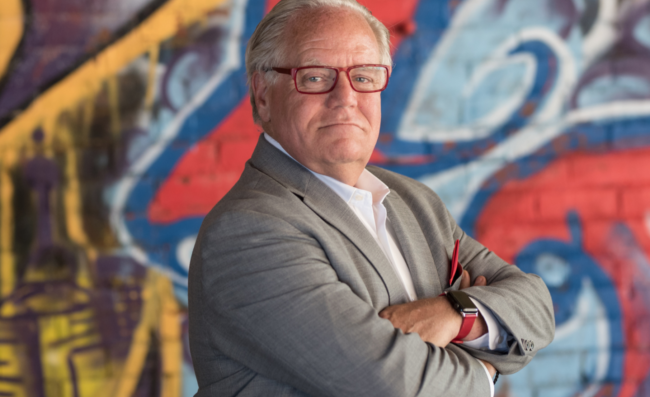
Jim Marous:
Hello! Welcome to Banking Transformed. I'm your host, Jim Marous, owner, CEO of Digital Bank Report to co-publish a financial brand. You've heard it from me before, you'll hear it from me again, there is nothing more important to digital transformation than data analytics, and the deployment of insights for the benefit of the customer. More than ever before, these advanced analytics, and these tools, are going to be a major differentiator in the financial marketplace as you compete with other financial institutions with Fintechs, and with the big tech firms. But the good news is, no matter what size you are, this is achievable.
Jim Marous:
On today's show, we have Nate Shahan, co-founder chief product officer of Segment. He will discuss how traditional finance institutes can leverage this high level data and insight capability to provide differentiation in the marketplace. There's never been a time when data and advanced analytics was more important for banking. From the development of new services, creating better experiences, or securing identity, banking needs smarter data faster. The key is to find ways to compress the timeline from transaction, to insights, to decisioning, to experience, the strategic advantage, and differentiation in the marketplace. To achieve the goal of speed of insights, most organizations are partnering with solution providers that are skilled at working with financial institutions. As I've mentioned, I have Nate Shahan, co-founder and chief product officer segment with me on the show today. Welcome to the show Nate. Before we start, could you provide me a little bit of insights into the history of Segment?
Nate Shahan:
Yeah, no problem. First, Jim, I've seen you speak many times. A big fan, and it's great to have an opportunity to sit down and chat with you over an important topic like this. I think one, we're both passionate about, and one Segment's been passionate about since the beginning. We've been around as an organization for over 14 years, and have really been all about helping financial institutions get more out of their data assets. It's really been our mission since day one. It's not about presenting those in a way that, in the form of reports, or analysis, as you would traditionally think. Our form of helping an institution get more out of data is also connecting that to ways to get action out of it, and deploy it in meaningful ways, but also scalable ways, to impact decisions and experiences that happen right now at the institution. That's where the industry's going. That's where the advancement of the topics we're talking about today is heading. That'll remain our mission going forward.
Jim Marous:
Well, it's interesting. We talked a little bit about it at the beginning, I've known your firm since it's beginning, because I'm local. I'm in Cleveland, you're in Akron. One of the interesting dynamics is that your firm has really transformed itself. Initially, you processed data. You really looked at data and said, "Here's the insights you can delve out of this," but you found, I think, if I get it right, that organizations, and they got your answers, but they didn't do anything with them. One of the things that I remember Segment doing very well, was really building marketing programs around your data. How important is that? The whole dynamic of your partnerships with financial institutions, being able to take data, analyze the data, and actually provide organizations with ideas that they can make money on the data processing you do.
Nate Shahan:
That's so key. So many of our clients and partners, especially from a marketing perspective, and an engagement perspective, so many of them are just like everyone else in the world. Low resources, low staff, minimum technology investments and capabilities. You could produce the best insights and intelligence about their customers, or members, for a large majority of the market and hand it over to them, but that really doesn't cover the last mile to the customer. We believed, early on, that we not only had to create intelligence, and insights, and meaningful understanding a customer, not just out of data at the institution, but the most difficult data available to work with at the institution, which is that understanding of spend and transaction analysis.
Nate Shahan:
We felt day one that we had to offer an automated way. Once those insights are derived very quickly, from the movement of that data, day over day, or real time, as we're receiving that data, to deploy it instantly through automated capabilities around customer engagement. Those small staffs, those limited resource teams within our client base can really benefit from the things that the giants in the industry are doing around data and engagement, we felt it was necessary to deploy that capability. Turnkey allowed them to engage with their customers as they're interacting on digital channels. They've made these massive digital investments, to expose to their customers, whether that be digital, mobile banking, customer service applications, chat box, or whatever the case may be, they've made these massive investments. They're getting great data out of it, and so use those interactions most effectively. We deploy our data with real use cases behind them.
Jim Marous:
You mentioned engagement. This has been a key element of things I've talked about recently. What do you mean when you talk about engagement? A lot of times we talk about how we can deploy data, make better experiences. We talk about it in very non-defined ways, but when you talk about engagement, what are you talking about, and what are you trying to deliver to your clients so that they can deliver to their clients?
Nate Shahan:
Engagement to us is every potential interaction that a customer, or account holder member, whatever the case may be, every single possible touch point they have with an institution. That crosses the board from, yes, most definitely, those digital channel engagements and interactions, the automation around onboarding solutions and applications for lending, but even those face to face interactions, those assisted, or in person interactions that a customer has with a teller, the drive-through banking, ATM, or whatever the case may be, that is an opportunity to personalize the experience. The institution has everything at their fingertips to make that experience personal. There's so many clues within the data that our client, the customers of the institutions, are providing the institution through leveraging their banking products, utilizing their card products, or their digital engagement platforms. There's so much valuable data there to personalize that interaction and that experience in a meaningful way. That's what we mean, is getting the data and the recommendations to really make those experiences as personal and meaningful as possible to the front lines, really covering that last mile between data and customer.
Jim Marous:
Boy, that's a great description because it really, it's more than just the personalization. It's actually trying to determine the journey that a person has to take to get to their financial goals. It's getting easier and easier. How do you describe AI and machine learning being used today in the financial services space? How does that differ to what it was used, maybe even two or three years ago?
Nate Shahan:
Yeah. I think it's very common just for anybody that's in the industry, our heads to go to, when we think about AI and machine learning, go to the applications of that around topics like business process automation, or customer service automation and applications, Alexa implementations, for example, or chatbot implementations, which all of those are great uses of the technology, but are really just scratching the surface in the ways that AI and ML solutions can and should be deployed. Really, just the start of the expanded use, I think, of the technology that we'll see in the coming years.
Jim Marous:
What feature does a good AI modeling platform actually entail when you're talking about a customer experience, as opposed to the security or risk assessments?
Nate Shahan:
Data is being produced in droves by account holders, customers and members of the institution, more so than we've seen in history. Tomorrow, we'll say it was produced more than it was yesterday. A lot of that is largely in part of a great adoption of technology and banking products, specifically digital banking solutions and automated banking solutions that are made available to our customers at the institution. Ultimately, though, the results of using those are producing more data than ever before, and in many cases, poor quality of data. When you mix new tech with legacy systems, the output of that is oftentimes very difficult to work with data, but very valuable data. Traditionally, AI modeling approaches, I would say oftentimes, span several weeks or months to get value out of them, specifically around leveraging ML for predictive modeling purposes.
Nate Shahan:
Traditionally, there was that step of data acquisition, figuring out what data assets are critical for a prediction about a customer. Actually building the model against that data requires clean and rich data. Usually, once you identify the data assets, they're very difficult to work with, or oftentimes difficult to understand. You have to prepare that data once you get it to really be useful to the model itself. Then you test the model, optimize the model, deploy it in the market, and then you go through the same rigorous approach of collecting data again, to see how the model performed. That is, don't get me wrong, a useful approach. For many uses and needs within the institution, still viable and necessary, but a good AI platform and approach needs to also offer a faster moving solution. Something that moves as quick as the data is moving and being produced by the customers and account holders.
Nate Shahan:
What you want to do in those cases is deploy a platform that has automated approaches to data enrichment, because again, the data quality that's being produced in volumes is not the best, specifically, when you look at transaction level data. It's oftentimes, once it lands within the core of the institution, in a state that's very difficult to work with and understand. It needs enrichment. Automation around data enrichment, and then automation around model predictions, and the ability to deploy those audiences that are derived from those predictions, to the channels and syndicate that data to the channels that our customers are interacting with in a very automated way, is all key. The big picture here is automation around AI, and being able to instantly detect how it's going, and automate the optimization process.
Jim Marous:
You know what's interesting? Financial institutions, there's not one that doesn't have a ton of data. The challenge is, almost every financial institution knows that they're not using it the best way, it's certainly not structured the right way, and it certainly isn't being used and deployed the right way. But except for the top, I'll say 10, but probably in the top five for financial institutions, the skill sets to make data actually actionable and showing how to take action is really a weakness. What should a financial institution look for when they're looking for a vendor, number one? And number two, do they have to wait until their data is really in good shape, or should a vendor be able to help them get there?
Nate Shahan:
That's a great question. Specifically, the second part of that question. We've seen over the years, I guess, the habit, or desire, to really contain the problem. What I mean by that is because there's so much data, we saw over the last five to 10 years a big push by many financial institutions to feel like, "Hey, I have to contain my data in a location. At least get it organized through data warehousing solutions, data lakes, data lake houses." Tomorrow it'll be called something else. Those are meaningful tasks, and produce a lot of value for the institution, but is not necessarily a prerequisite that has to occur before getting value out of this data that's flowing through the institution. What I would look for, or recommend for anybody looking to take meaningful steps in this direction, and are evaluating vendors, is really dig into the data that the vendor will utilize in their approach.
Nate Shahan:
It's specifically the data assets that they're looking to consume within their process, and their technology. Traditional approaches around AI predictions, predictive modeling, and so on, really rely on slower moving data signals, things like month over month balance changes, or what were the number of branch visits that occurred, or teller interactions that occurred. All useful data in making predictions, but modern techniques really thrive on what we consider being the most valuable data produced by account holders in large volumes, which things like spend level data. The actual transactions that are occurring on these accounts with specific merchants, or competing financial institutions, as well as some of that channel interaction data. The signals that are available through how we behave on those digital experiences that are being consumed by the account holder.
Nate Shahan:
Really digging into the data assets that are being utilized in the process, they very much should include those difficult to work with data assets that occur in volume, like transaction level data. I would ensure that they're consuming that type of data in their predictions and in their process. I would ensure that they have automated approaches to distilling that data into something meaningful and useful very quickly, as it occurs. Three months is too long to analyze and enrich a transaction to prepare it for a model. You got three minutes. Make it work right now. Those are two key areas that I would focus on, from a vendor assessment perspective.
Jim Marous:
From your perspective, but also things I've seen, you keep on referencing speed. I think organizations really underestimate the importance and the prioritization of speed, not just speed from data analytics to action or deployment, but speed of the decision to select a vendor. We used to have a luxury of taking 12 to 18 months to select a vendor for anything we wanted to do in the financial service industry. That luxury is gone. In fact, the risk of taking that amount of time is greater than the risk making a wrong decision. I'm familiar with a lot of the players in the marketplace that you work. They're all really good players. It is better to make an imperfect decision today and change it, than to try to make the perfect decision 12 months from now, only to find that that decision probably is different if you make it six months from then.
Jim Marous:
Find the partner, find somebody you're comfortable with, and make the decision today to move forward, because honestly, in the right traditional financial services industry, right now, the amount of time that you wait to do something is killing you. The reality, and you talk about even the decisions around what you do with the data you get, I reference often, a GPS system, that if you're getting data back from your GPS system every hour, as opposed to every minute, you could be 60 miles away from the place you wanted to be and on a completely different route, if you're only getting data that frequently. People are now used to instantaneous ideas on what you should do proactively to avoid a problem in the future. That analogy of the GPS system works really well in financial services.
Jim Marous:
Because I don't want to know what's already happened with my account, as much as I want to know, what should I do tomorrow to make it so that my financial wellness is greater? Again, in one other thing I'd mentioned around picking a vendor is, pick a vendor that already has case studies. They can deploy almost instantaneously to bring you victories. I know that's one thing that your firm has really emphasized in the past is you have a lot of case studies on things that have worked. When an organization's making a decision to find a vendor, and they need to justify that decision rather quickly, it's good to have those case studies in your back pocket to say, "We've done this, this, this, and this already. We need more funding to go even further." Again, reinforcing what say, but it's interesting because in speaking with financial institutions, they often are hesitant to engage in AI modeling because they see it as a security risk around their data. What does a good vendor do to mitigate this risk around data utilization?
Nate Shahan:
It's a valid concern. It should be top of mind anytime there's an initiative around the communication of data, or exposing data to a vendor for use in AI and ML practices. It should be. It should remain that way. It should always be top of mind. The thing I want to point out, first and foremost, is there's really no substitute for a well implemented and rigorous security and controls program around data. Even if a vendor isn't collecting what is maybe considered sensitive, or personally identifiable information from the institution, they should have controls in place as if they do. Vendors should really care for the data as rigorously, and with as much discipline, as a financial institution does themselves. Take them through that process and ensure that that fundamental is there.
Nate Shahan:
Even when that is there, when those capabilities are there with the vendor, it shouldn't be a license to deploy personally identifiable information everywhere. What I mean by that, is if the job can be done, if a prediction can be made without the need to share personally identifiable information, then you shouldn't share it. There's no need to do that with today's modern technology. Why put PII and sensitive data in harm's way if it really isn't necessary to the process, or the use case, in the first place? But if PII is necessary and key to the strategy, then consider a vendor that is utilizing ways to obfuscate and protect that personally identifiable information through what we consider modern approaches. One of those is through tokenization of that data. Ensure that they have the ability and technologies in place to tokenize that data if it must flow into their technology ecosystem. We recently announced a partnership with who we consider, not just in FinTech, but just the technology industry in general, a company called TokenEx, whose entire business is centered around the ability to tokenize sensitive data and let it flow through innovation and technology ecosystems in a very efficient way. Make sure that that vendor offers at least the capability to tokenize that sensitive information.
Nate Shahan:
The last thing I'd look for is to ensure that the vendor offers capabilities, where those capabilities can be brought to the data. Where it resides within the institution and their cloud environments today, in a situation where, either due to large volumes of data, or the sensitivity of data, is probably best not to leave the walls of the institution. Work with a vendor who's using, again, modern technologies that allow their platforms, their analysis, their predictions, their models, to run in the controlled environment of the financial institution. Which is, you may have seen recently, we announced a partnership with Snowflake. We're building our analytics capabilities on that platform for just this purpose and reason. High volumes of data, very difficult to copy, and share, and move, even in a Cloud-based world that we in. There's a lot of sensitivity around spend information, and how people are spending their money, that should remain private. Bring the technology to the data using capabilities like Snowflake, or similar platforms.
Jim Marous:
Essentially, throughout your whole history as an organization, you've really been focused on what I'm going to call the next level of data. More than just balances and things in this nature, you've really been focused on transaction data, which is just massive amounts of volume, but it's sometimes hard to get your arms around. Why is transaction data so important to AI modeling, but even more so toward the solutions that you implement in the marketplace?
Nate Shahan:
When we say transaction data, historically, the definition of transaction data, specifically a modeling, has been a transaction is a deposit with a teller, or a drive-through interaction at the drive-through window at the institution. That has been, historically, what's been described as a transaction. Again, that kind of data, the slower moving data that I described earlier, that has been useful in predictive modeling, and in some of the more traditional approaches. Still very useful data, valuable data. It's not bad by any means. What's very valuable, and really telling, and really helps an institution achieve an understanding of what's going on in their account holder's life, you have to follow the money. Where are their spending behaviors? Who they occur with? How often they occur? Understand the patterns of that purchase behavior, and the brands that they engage with on a regular basis.
Nate Shahan:
It's been very difficult, historically, to utilize that data in predictions, or customer, understanding because of the quality of that data. Once it lands within the core systems of the financial institution, the merchant information that's available, really understanding where the purchase took place, that data has been truncated, manipulated multiple times through the payment's ecosystem, from swipe to core, that it's, in many cases, a complete mess at that point in time. It's been very difficult to leverage that data in these predictions, because it is produced in such high volumes and poor quality. What's unique about the approach that we've brought to market is the ability to distill that information, enrich it, and really tag it with supplemental data to make it more useful for not just a machine, but also human. In order to get a real understanding about what's going on in someone's life, you have to understand where and why the money's moving. That's really the key driver between that last mile of just having the data available, and putting it to use in a very personalized way. For us, it's not a nice to have, it's a must have, for financial institutions in the future.
Jim Marous:
Getting down to the really core of it all, how is data AI and machine learning a differentiator that a financial institution can implement against their competition?
Nate Shahan:
Again, I kind of go back to a previous conversation you and I had, which was around a lot of the implementations of AI and ML solutions, maybe oversimplifying it a bit here, but really have been siloed, and for the benefit of the institution, more so than the end customer. What I mean by that is if you look at traditional implementations around very important topics like risk or fraud detection, or even automation of business processes, those are very useful applications and very important applications, but oftentimes the benefits of those are transparent to the end customer. It really benefits the financial institution. I think the reason for that is because AI and ML haven't yet, in the next frontier, been deployed effectively across the institution to understand data.
Nate Shahan:
That's really the next step for the industry. Really, the technology's there. Modern technology is in place to allow an institution of any size first and foremost, to understand their data, and understand their customer with AI and ML solutions, vendors, and partnerships, and practices, to really forge ahead on innovation. Differentiator innovation that'll really change the lives of the customer, and will be obvious to them to really secure that relationship, not only to acquire that relationship, but secure it for the long term, and build that lasting relationship with customer.
Jim Marous:
That is so key. We've talked about on other podcasts, I've written about it recently is that, as opposed to simply driving a marketing communication, good use of data, AI and machine learning, should also drive innovation, product development, and you reference it. If you deployed across the entire organization, think about the power of your tellers being able to reach out to customers that no longer see, they don't come in the branch anymore, but they can reach out to them and say, "You know what, based on what you've done recently, we think this may be a good idea for you." This is a kind of relationship that Amazon has with their customers. It's kind of relationship that Netflix has with me and picking what I want to watch next. I'm just a relatively new Netflix customer, but I am amazed how Netflix, Hulu, all these others, can really define in a very short period of time, what I'd be interested in next.
Jim Marous:
Well, because of these interactions with other industries, consumers more than ever are not going to be forgiving if a teller doesn't understand the journey they're taking, if a product manager doesn't understand the journey they're taking, or worse yet, if a marketing communication piece takes the most finite details and avoids them. I have a situation with my current financial institution where they keep on reaching out to me and saying, "You should be making sure you take advantage of mobile banking, and also remote deposit cash." I'm going, "I have both," but what's happened is somewhere in the legal part of the organization, they're saying we don't want to make a mistake by misidentifying. Well, you know what? You're making a bigger mistake by not identifying. I think you're right. The differentiation in financial institutions is going to be all based on data, but not on how you're doing it well for protecting me, but for knowing me, understanding me, and rewarding me for my relationship in much the same way that Spotify, Apple, Amazon, and others do.
Jim Marous:
Thanks for listening to Banking Transform, rated as a top five banking podcast and winner of three international awards for podcast excellence. If you enjoy what we're doing, please take a little bit of time and give us a rating so we can get more guests like Nate on the show. In addition, if you're a financial institution that wants to be interviewed as part of our bank and transformed solution podcast, reach out to me and we'll see if we can't work something out. Finally, be sure to catch my recent articles on the financial brand and check out the research we're doing for the digital banking report.
Jim Marous:
This has been a production of Evergreen podcast. A special thank you to a producer, Leah Longbrake, audio engineer, Sean Rule-Hoffman, and video producer Will Pritts. I'm your host, Jim Marous. Until next time, remember, don't wait to do the important things. Do them now.